What is Generative AI? – Meaning and Definition
Generative AI is a powerful technology that can learn from existing data and create new, realistic content on a large scale without just repeating what it’s seen before. It can make all sorts of things like images, videos, music, text, and more.
Generative AI relies on special AI models that learn from lots of data. These models are like versatile tools that can be customized for different jobs. Making these models is complex and needs a lot of computer power, but it’s kind of like teaching them to predict things.
Right now, one of the common ways we use generative AI is to make things when people ask for them in regular language. You don’t need to know how to code to use it. But this technology has lots of potential in the business world too, like helping design new drugs, chips, and materials, as mentioned in the Gartner report.
What is Generative AI? – Meaning and Definition
A growing number of breakthrough in natural language understanding.
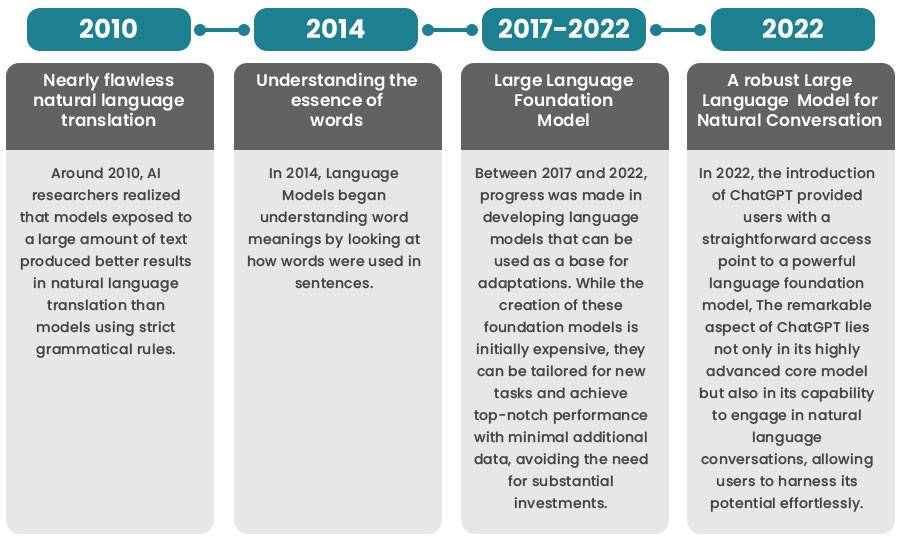
How Does Generative AI Work?
The field of generative AI is truly captivating, employing a range of methodologies such as neural networks and deep learning algorithms to discern patterns and produce novel results. In essence, it mimics the creative processes of the human mind through artificial intelligence.
Generative AI leverages a multitude of methods, encompassing neural networks and deep learning algorithms, to recognize patterns and produce novel outcomes rooted in these patterns.
In the training phase of a generative model, an extensive dataset comprising examples like images, text, audio, and videos is provided as input. The model subsequently scrutinizes the patterns and correlations embedded within this data, seeking to decipher the fundamental principles governing the content. To create fresh data, it samples from a probability distribution it has acquired through learning. Furthermore, it consistently fine-tunes its parameters to optimize the likelihood of producing precise outputs.
As an illustration, consider a generative model that has been trained on a collection of cat images. This model can be employed to generate novel cat images by drawing from the acquired distribution and subsequently improving the output using a technique known as “inference.” In the process of inference, the model fine-tunes its output to more closely resemble the intended result or rectify any inaccuracies. This guarantees that the generated output progressively becomes more lifelike and aligns more effectively with the user’s preferences.
How to Evaluate Generative AI Models?
Three essential prerequisites for a successful generative AI model include:
Quality: Quality is paramount, particularly in applications involving direct user interaction. For instance, in speech generation, maintaining a high standard of speech clarity is crucial for comprehension. Likewise, in image generation, the intended outputs should possess visual fidelity to the extent that they are virtually indistinguishable from natural images.
Diversity: Diversity is essential, as an effective generative model should encompass the less common patterns within its data distribution while preserving the quality of its generated content. This approach contributes to the mitigation of undesirable biases within the learned models.
Speed: Speed is a critical factor, particularly in interactive applications where swift generation is necessary, such as real-time image editing, to facilitate integration into content creation workflows.
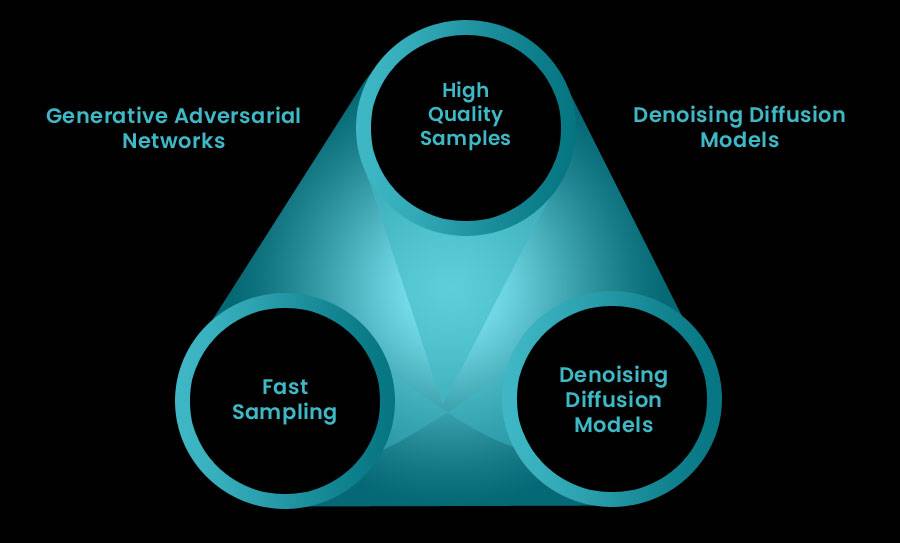
How to Develop Generative AI Models?
There are different types of generative models that offer unique strengths, and when you combine these strengths, you can create more powerful models.
Here’s a detailed breakdown:
Diffusion models: Diffusion models, sometimes referred to as denoising diffusion probabilistic models (DDPMs), are a type of generative model. They work through a two-step process during training, involving forward diffusion and reverse diffusion. In the forward diffusion step, random noise is gradually added to the training data, and in the reverse diffusion step, this noise is undone to reconstruct the data samples. To generate new data, one can initiate the reverse denoising process from completely random noise.
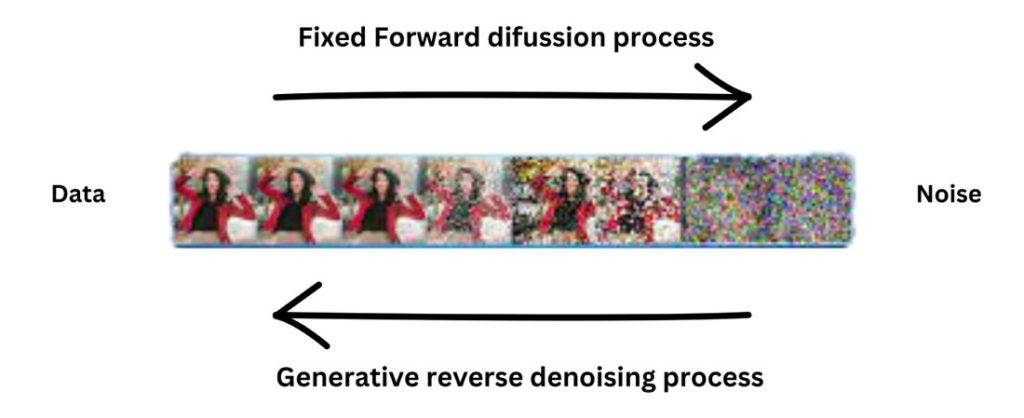
While training a diffusion model might require more time compared to a variational autoencoder (VAE), the advantage lies in its ability to train hundreds or even an unlimited number of layers. This attribute typically results in diffusion models delivering superior output quality when constructing generative AI models.
Furthermore, diffusion models fall into the category of foundational models due to their expansive scale, capacity to produce high-quality outputs, adaptability, and suitability for a wide range of applications. Nevertheless, their reverse sampling process makes running foundational models a time-consuming and protracted endeavor.
Explore the mathematical aspects of diffusion models in this blog post
Variational autoencoders (VAEs): VAEs comprise two neural networks, commonly known as the encoder and decoder. When provided with an input, the encoder transforms it into a more compact and dense data representation. This condensed representation retains the necessary information for the decoder to recreate the original input data, eliminating any extraneous details. The encoder and decoder collaborate to acquire an efficient and simplified latent data representation. This facilitates the generation of new latent representations that can be passed through the decoder to produce innovative data.
Although VAEs can generate outputs like images more rapidly, the images they produce lack the level of detail found in diffusion models.
Generative adversarial networks (GANs): Unveiled in 2014, GANs were the prevailing methodology among the three until the recent emergence of diffusion models. GANs engage in a duel between two neural networks: a generator, responsible for creating new instances, and a discriminator, tasked with becoming proficient in distinguishing the generated content as either authentic (from the domain) or counterfeit.
These two models are trained in tandem and become more proficient as the generator produces higher-quality content, while the discriminator becomes more adept at identifying generated material. This iterative process continues, compelling both models to constantly refine themselves after each cycle until the generated content becomes indiscernible from the original content.
Although GANs can swiftly generate high-quality samples, they often exhibit limited sample diversity, making them particularly suitable for generating data within specific domains.
Another crucial aspect in the advancement of generative models is the underlying architecture, with the transformer network being among the most widely used. It’s essential to grasp its functioning within the realm of generative AI.
Transformer networks, akin to recurrent neural networks, are engineered to handle sequential input data without relying on sequential processing.
Transformers excel in text-based generative AI applications due to two key mechanisms: self attention and positional encodings. These technologies collectively enable the algorithm to capture temporal relationships and facilitate long-range interactions between words.
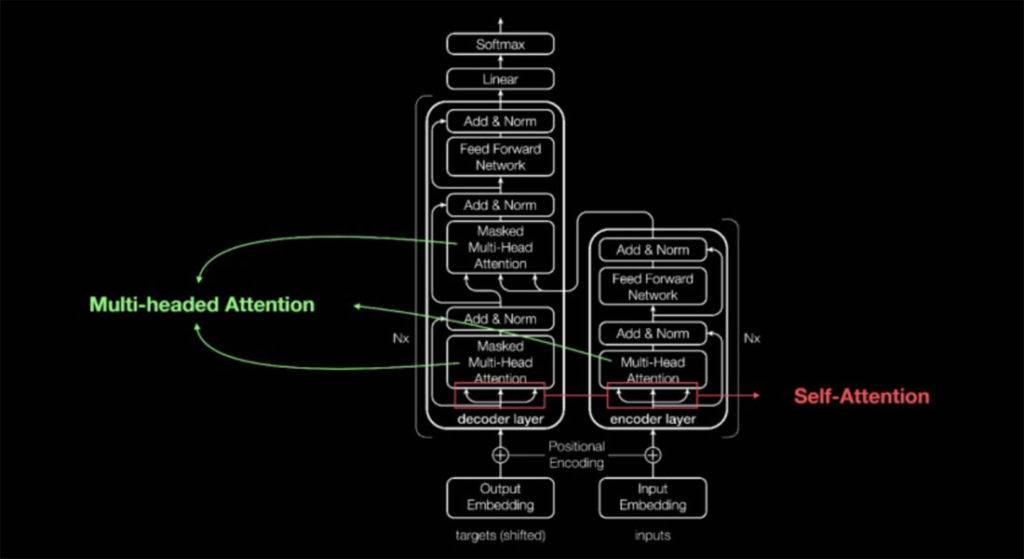
A self-attention layer is responsible for assigning significance weights to different elements within an input. These weights indicate how crucial each element is within the context of the entire input. On the other hand, positional encoding serves as a representation that captures the sequential order or positions of words within the input.
A transformer architecture comprises multiple transformer blocks, often referred to as layers. These transformer blocks consist of self-attention layers, feed-forward layers, and normalization layers, all collaborating to interpret and make predictions on sequences of tokenized data. These data sequences can encompass various types of information, such as text, protein sequences, or even image patches.
What are the Applications of Generative AI?
Generative AI serves as a potent tool for enhancing the productivity of various professionals, including creatives, engineers, researchers, scientists, and more. Its applications and potential are extensive, encompassing a wide range of industries and individuals.
Generative AI models have the capacity to accept inputs in various forms, including text, images, audio, video, and code, and produce new content in any of these modalities. For instance, they can transform text inputs into images, convert images into music, or transcribe video into text.
Below are some of the most widely used applications of generative AI:
Language: Text serves as the foundational element for numerous generative AI models, and it is widely regarded as the most sophisticated domain in this field. Among the prominent instances of generative models based on language, we find what are commonly referred to as large language models (LLMs). These expansive language models are currently being applied across a diverse range of tasks, encompassing activities such as generating essays, facilitating code development, enabling translation services, and even aiding in the comprehension of genetic sequences.
Audio: Generative AI is extending its reach into the realms of music, audio, and speech, marking the emergence of exciting frontiers. Notable instances encompass models capable of composing songs and crafting audio snippets based on textual inputs, identifying objects in videos while generating corresponding sounds for diverse video content, and even producing personalized music compositions.
Visual: Generative AI finds widespread popularity in the domain of visual content, enabling the generation of various forms of imagery such as 3D visuals, avatars, videos, graphs, and other visual representations. It offers the versatility to produce images with diverse aesthetic styles and provides tools for editing and adapting the generated visuals. Generative AI models prove invaluable in tasks like generating graphs depicting novel chemical compounds and molecules for advancements in drug discovery, crafting lifelike visuals for virtual or augmented reality experiences, developing 3D models for video games, designing logos, enhancing or altering existing images, and a host of other creative applications.
Synthetic Data: The utilization of synthetic data is of paramount importance in training AI models, particularly in scenarios where data is absent, constrained, or incapable of handling edge cases with utmost precision. The generation of synthetic data using generative models stands out as a highly influential remedy for addressing the data-related challenges faced by numerous enterprises. This approach is applicable across a wide spectrum of modalities and use cases and is made feasible through a technique known as label-efficient learning. Generative AI models play a pivotal role in mitigating labeling expenses by either autonomously generating additional augmented training data or by acquiring an internal representation of the data that streamlines the training of AI models with reduced reliance on labeled data.
The influence of generative models is vast and continually expanding, with their applications making significant contributions to the progression and evolution of domains such as transportation, natural sciences, and entertainment.
Within the automotive sector, the utilization of generative AI is poised to play a pivotal role in the generation of 3D environments and models for simulations and the advancement of car development processes. Synthetic data is also being harnessed to train autonomous vehicles. This capability to conduct road tests in a lifelike 3D environment enhances safety, efficiency, and adaptability while concurrently reducing risks and operational costs.
Generative AI exerts a profound impact on the realm of natural sciences, offering valuable contributions across various domains. In the healthcare sector, these generative models prove instrumental in medical research by generating novel protein sequences that facilitate drug discovery. Additionally, practitioners benefit from the automation of tasks like transcription, medical coding, medical imaging analysis, and genomic research. Meanwhile, in meteorology, generative models find utility in crafting simulations of the Earth, aiding in precise weather predictions and the anticipation of natural disasters. These applications collectively contribute to the creation of safer environments for the general populace while empowering scientists to predict and prepare more effectively for natural calamities.
The entertainment industry, spanning video games, film, animation, world-building, and virtual reality, harnesses generative AI models to streamline content creation across all its facets. Creators view these models as valuable tools that complement their creative endeavors and facilitate their work.
What are the Challenges of Generative AI?
Artificial intelligence (AI) has ushered in significant changes across various domains in recent years. Among the various AI technologies, Generative AI has emerged as a crucial component in numerous industries, offering a wide array of benefits. At its core, Generative AI comprises programs that enable machines to employ specific algorithms for the creation of new content resembling the original resources, which can include images, text, audio files, and code. This technology holds immense promise for enhancing decision-making processes and fostering innovation.
Despite its growing importance and contributions across diverse sectors, the adoption of Generative AI also presents several challenges. What are some of the obstacles associated with the integration of Generative AI?
The challenges of the application of Generative AI include
Control Limitation: Generative AI methods, like Generative Adversarial Networks (GANs), can exhibit unpredictability and instability in specific circumstances, resulting in unexpected outputs without clear explanations. Consequently, identifying appropriate solutions for these issues can be challenging.
Pseudo Image Generation: Generative AI algorithms require substantial amounts of data to complete tasks but are unable to produce entirely novel images. Instead, they generate images by recombining the information they’ve gathered in unique ways.
Security Issue: The use of Generative AI has the potential to contribute to a rise in identity theft, fraud, and counterfeiting incidents because it can generate fake photos and images that closely mimic genuine ones.
Data Privacy Concern: The utilization of Generative AI in healthcare may raise concerns about data privacy, as it entails the collection of individuals’ sensitive information.
Ethics and religions perspective issue: Similar to cloning technology, the use of Generative AI can raise moral concerns in society. For example, Generative AI’s ability to transform human faces into the opposite gender through generated photos could become a sensitive matter for specific religious groups. Therefore, when exploring the potential of Generative AI, it is essential to establish guidelines for innovation that consider ethical and religious perspectives, particularly when the technology has the potential to introduce social, ethical, and religious dilemmas.
What are the Benefits of Generative AI?
Generative AI holds significance for various reasons, and it offers several key advantages, including:
Generative AI algorithms have the capability to produce fresh and authentic content, including images, videos, and text, that is virtually indistinguishable from human-created content. This quality can find valuable applications in areas like entertainment, advertising, and the creative arts.
Generative AI algorithms have the potential to enhance the effectiveness and precision of current AI systems, such as those used in natural language processing and computer vision. One application involves generating synthetic data to train and assess other AI algorithms, thereby improving their performance.
Generative AI algorithms enable the exploration and analysis of intricate data from fresh perspectives. This capability empowers businesses and researchers to reveal concealed patterns and trends that might remain hidden when examining the raw data alone.
Generative AI algorithms can assist in the automation and acceleration of various tasks and processes, leading to time and resource savings for businesses and organizations.
Conclusion:
In summary, the advantages of generative AI are vast and far-reaching. This technology holds the promise of benefiting humanity in numerous ways, such as stimulating innovation and creativity, enhancing productivity and efficiency, expanding available resources, and refining personalization and customization. With continued advancements in this field, we can look forward to witnessing even more captivating progress and discoveries.