In the evolving landscape of healthcare, the infusion of cutting-edge technologies promises to reshape our understanding and approach towards diagnosis, treatment, and research. Among the many technological marvels, Generative AI stands out as an avant-garde player, offering transformative capabilities that were once considered the realm of science fiction.
In the healthcare domain, Generative AI’s primary allure lies in its ability to generate synthetic data, which can have vast implications for various facets of medical research and patient care.
With the potential to unlock new avenues in healthcare, the inclusion of generative methodologies can be a game-changer.
For instance, consider the world of medical imaging. By generating high-quality, synthetic images, Generative AI can augment datasets, enabling researchers and clinicians to work with a broader array of data without needing to access new real-world samples. Such augmentation can potentially lead to the development of improved diagnostic tools and techniques.
Similarly, in the realm of genetic research, the generation of synthetic DNA sequences can pave the way for a deeper understanding of genetic disorders, even those that are rare and less understood.
Generative AI can also play a pivotal role in simulation-based training and education for medical professionals. By creating realistic, synthetic patient scenarios, it can provide an invaluable tool for training without risking real patient well-being.
Moreover, the world of drug development, often hindered by long trial periods and immense costs, can be revolutionized by Generative AI. Imagine simulating how synthetic molecules might interact with human tissues or predicting how a new drug formulation might behave without first producing it.
Beyond these examples, the promise of Generative AI in healthcare lies in its versatility and adaptability. As algorithms grow more sophisticated and as we continue integrating them into healthcare systems, we might only be scratching the surface of what’s possible.
While the above provides a glimpse into the potential of Generative AI in healthcare, it’s a vast and rapidly evolving field. It’s crucial to stay updated and explore its numerous facets to truly appreciate its transformative power and the future it’s crafting for healthcare.
Highlighting the difference between Traditional and Generative AI in Healthcare
The integration of artificial intelligence in healthcare has been nothing short of transformative. As we navigate through the AI spectrum in medicine, it becomes essential to distinguish between traditional AI and generative AI, two powerhouses with distinct approaches and utilities. Let’s delve into their differences in the healthcare context:
Purpose and Functionality: Traditional AI is oriented towards tasks such as classification, prediction, and decision-making. In healthcare, this could involve predicting the likelihood of a disease based on patient data. Generative AI, on the other hand, focuses on crafting synthetic data like realistic medical images not tied to an actual patient or fabricating DNA sequences for research.
Data Handling: Traditional models rely on pre-existing data for analysis, with accuracy being directly influenced by the quality of this data. In contrast, generative AI, while starting with an initial dataset, emphasizes generating novel data that augments the potential data pool.
Application Scenarios: Traditional approaches have been instrumental in tasks like predicting patient readmission rates and aiding in diagnosis through image recognition. Generative AI has broader applications, such as creating synthetic patient datasets without compromising privacy, propelling drug discovery by generating new molecular structures, and simulating patient responses for medical training.
Dependency on Training Data: The effectiveness of traditional AI is tied to the volume and quality of training data, and any inherent bias can skew outputs. Generative AI, although also relying on initial training data, mitigates some limitations tied to data scarcity or a lack of diversity by generating new datasets.
Outcome Variability: Traditional models are deterministic, generally producing the same output for the same input. In contrast, generative AI can produce a myriad of outputs for the same or similar inputs, highlighting its capacity for diversity.
Interpretability: While many traditional models offer a degree of transparency, allowing users to comprehend the logic behind predictions, generative AI, especially deep models, tend to be more intricate. Their complexity can sometimes pose challenges to understanding their inner workings, which might be crucial in healthcare scenarios.
Various Generative AI Models Applied to the Healthcare Industry
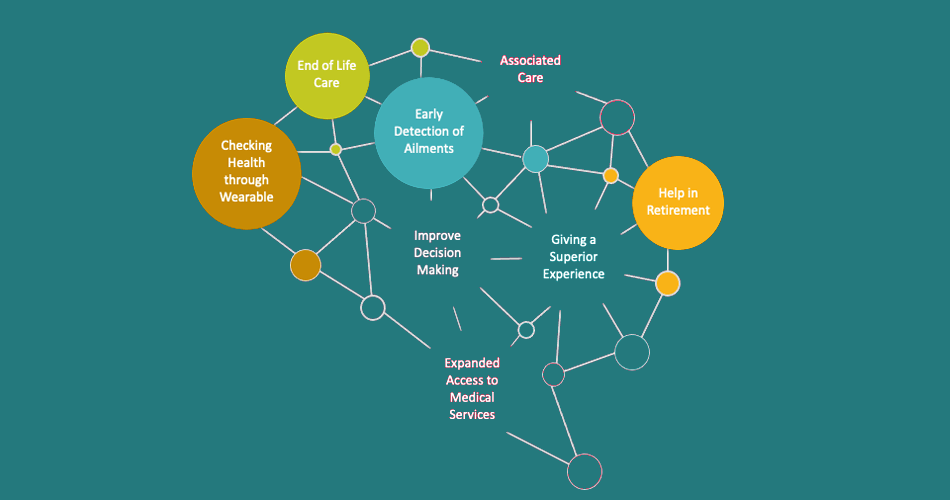
The advent of Generative AI has presented a range of models that have made significant strides in the healthcare domain. These models, with their capability to generate synthetic data, have empowered researchers, clinicians, and healthcare professionals to dive deeper into diagnostics, drug discovery, personalized treatment, and more. Within the vast landscape of generative AI in healthcare, several generative model types have been making significant waves:
Generative Adversarial Networks (GANs): These are composed of two competing neural networks: a generator that crafts synthetic data and a discriminator that discerns between genuine and synthesized data. Over multiple iterations, the generator refines its capabilities, creating data that’s increasingly challenging for the discriminator to differentiate. In healthcare, GANs play a pivotal role in synthesizing realistic data, such as enhancing medical images or crafting patient demographic datasets, which are instrumental in diagnostics and research.
Variational Autoencoders (VAEs): VAEs function through a dual mechanism: an encoder that morphs input data into a latent representation and a decoder that recreates data from this latent space. VAEs utilize a probabilistic model to generate varied data samples. In the healthcare domain, they aid in simulating diverse patient scenarios, mapping disease progressions, and spearheading advancements in drug discovery.
Restricted Boltzmann Machines (RBMs): As energy-based models, RBMs learn the probability distribution of their input data, effectively extracting essential features that can be utilized for diagnostics and personalized treatment plans.
Recurrent Neural Networks (RNNs): Recognized for their proficiency with sequential data, RNNs predict subsequent data points based on prior inputs, making them crucial for analyzing patient records, predicting disease trajectories, and assessing medical device data.
Transformer-based Generative Models: Leveraging self-attention mechanisms, these models excel in handling sequences, making them apt for predicting protein structures, understanding genetic sequences, or even auto-generating medical reports.
Molecular Generative Models: Tailored for drug discovery, these models are adept at predicting molecular structures, refining drug attributes, and conceptualizing innovative drug compounds.
In summation, the diverse range of generative models, from GANs and VAEs to transformerbased and molecular models, each offer unique data generation capabilities in healthcare. Their integration promises transformative changes, reshaping the landscape of diagnostics, treatments, and patient care in unprecedented ways.
These models represent just the tip of the iceberg when it comes to Generative AI in healthcare. As research progresses and the domain matures, it’s likely that newer, more specialized models will emerge, each catering to specific challenges and tasks within the healthcare industry. The confluence of Generative AI and healthcare holds the promise of revolutionizing diagnostics, treatments, research, and patient care in unprecedented ways.
Advantage of Generative AI in the Healthcare Industry
Generative AI, with its distinct ability to produce synthetic data and simulate various scenarios, has become a pivotal asset in the healthcare industry. The potential benefits it brings are manifold, revolutionizing various aspects of medical research, diagnosis, treatment, and patient care. Here’s an exploration of the myriad advantages offered by Generative AI in healthcare:
Enhanced Medical Imaging: Generative AI can produce high-quality, synthetic medical images, augmenting existing datasets. This enables researchers to work with a more comprehensive set of images without the need for new real-world samples, potentially leading to improved diagnostic tools and techniques.
Data Augmentation for Research: In instances where real medical data is scarce or lacks diversity, Generative AI can create supplementary synthetic data. This can be particularly useful in studying rare diseases or conditions that have limited available data.
Preservation of Patient Privacy: Generating synthetic patient data that doesn’t trace back to real individuals can be a boon for research. It provides valuable data for study and analysis without compromising patient confidentiality.
Drug Development and Discovery: Generative AI can accelerate the drug development process by simulating how synthetic molecules might interact with human tissues, predicting how new drug compounds might behave, or suggesting new molecular structures. This can reduce the time and cost of bringing a new drug to market.
Personalized Treatment Plans: By analyzing a patient’s medical history and simulating various treatment outcomes, Generative AI can assist in devising personalized treatment plans that are tailored for the individual’s unique needs.
Medical Training and Simulation: Generative AI can create realistic patient scenarios for medical professionals’ training. This provides a safe environment for medical students and professionals to learn and practice without risking real patient well-being.
Application of Generative AI in the Healthcare Industry
Generative AI’s capabilities have been harnessed across a broad spectrum of the healthcare sector, providing innovative solutions, driving research, and enhancing patient care. Here are some prominent applications of Generative AI in the healthcare industry:
Medical Imaging:
- Synthetic Image Generation: Generative AI models can produce synthetic medical images, which can be especially valuable for training machine learning models where real-world data might be limited.
- Image Augmentation: Improve the quality and resolution of medical images, aiding in more accurate diagnostics.
- Image-to-Image Translation: Convert one type of medical image into another (e.g., MRI to CT scan), providing additional perspectives without subjecting patients to multiple imaging procedures.
Drug Discovery:
- Molecule Generation: Design novel molecular structures for potential drugs, targeting specific diseases or conditions.
- Drug Property Prediction: Predict the properties or potential side effects of drug molecules, streamlining the drug development process.
- Drug Repurposing: Identify existing drugs that could be effective for other diseases based on their molecular structures and interactions.
Data Privacy and Research:
- Synthetic Health Records: Create realistic, but entirely synthetic, patient health records for research purposes without compromising patient privacy.
- Disease Modeling: Simulate the progression of diseases in synthetic populations to study epidemiology and potential interventions.
Personalized Medicine:
- Treatment Simulations: Use patient data to simulate different treatment outcomes, allowing for the optimization of personalized treatment plans.
- Disease Risk Prediction: Simulate the potential future health trajectory of patients based on their current health data and lifestyle.
Genetic Research:
- DNA Sequence Generation: Produce synthetic DNA sequences to aid in genetic research, potentially helping in understanding genetic disorders or exploring gene editing possibilities.
Medical Training:
- Patient Scenario Simulations: Train medical students and professionals by simulating realistic patient cases, enhancing their decision-making skills without real-world consequences.
Prosthetics and Bioprinting:
- Tissue and Organ Modeling: Use generative models to design 3D structures of tissues or organs, which can be utilized in bioprinting or prosthetic development.
Mental Health:
- Therapeutic Chatbots: Develop AI-driven chatbots that simulate therapeutic conversations, offering initial support or coping strategies to those in need.
Rehabilitation:
- Motion Prediction: Generative models can be used to predict and simulate natural human motions, aiding in the development of better rehabilitation protocols for patients recovering from injuries or surgeries.
Nutrition and Diet:
- Dietary Planning: Generate personalized dietary plans by simulating the effects of different nutrients and foods on an individual’s health.
Real World Example: How Healthcare Employ Generative AI
Enhancing Drug Discovery with Insilico Medicine:
- Context: Traditional drug discovery processes are time-consuming and expensive.
- Application: Insilico Medicine utilizes Generative Adversarial Networks. (GANs) to expedite the drug discovery process.
- Outcome: The company reported that their AI model designed, synthesized, and validated a novel drug candidate in just 46 days, drastically reducing the usual time.
Synthetic MRI Images with NVIDIA:
- Context: Acquiring a large dataset of medical images for training AI models can be challenging due to privacy concerns and data scarcity.
- Application: NVIDIA used GANs to generate synthetic brain MRI images, helping in training AI models for medical image analysis without the need for real patient data.
- Outcome: The synthetic images aid in the development and validation of neural network models in medical imaging.
Personalized Treatment Plans with Tempus:
- Context: Treatment plans often follow a generalized approach, which might not be optimal for all patients.
- Application: Tempus uses AI, including generative models, to analyze clinical data and devise personalized treatment plans, taking into account the unique genetic makeup of each patient.
- Outcome: The use of Generative AI aids in predicting patient responses to different treatments, optimizing therapy plans for better outcomes.
Early Disease Detection with PathAI:
- Context: Early detection of diseases can drastically improve patient outcomes, but spotting early-stage anomalies in medical images is challenging.
- Application: PathAI uses deep learning, including generative models, to analyze pathology slides. The system can detect minute abnormalities that might be overlooked by human eyes.
- Outcome: Improved accuracy and speed in diagnosing diseases, enabling timely interventions.
Drug Repurposing with BenevolentAI:
- Context: Developing new drugs is expensive. An alternative is to find new uses for existing drugs.
- Application: BenevolentAI employs Generative AI models to explore the vast space of molecular structures and known drug profiles to identify existing drugs that might be repurposed for treating other conditions.
- Outcome: The company has identified potential new treatments for diseases, including Parkinson’s and COVID-19, using existing drugs, which can reduce the time and cost of introducing these treatments to patients.
As the intersection of Generative AI and healthcare continues to evolve, there’s immense potential for transformative shifts in various domains of medical science and patient care.
Market Growth and Forecast: The dynamism in this field is reflected by the anticipated market size for Generative AI in healthcare. From a valuation of USD 0.8 Bn in 2022, the Generative AI in Healthcare Market size is projected to skyrocket to around USD 17.2 Bn by 2032. This suggests a compound annual growth rate (CAGR) of 37.0% during the forecast period from 2023 to 2032, underscoring the tremendous potential and growing trust in this technology within the healthcare sector.
Prospect of Generative AI in Healthcare
As the intersection of Generative AI and healthcare continues to evolve, there’s immense potential for transformative shifts in various domains of medical science and patient care. Below are some prospects of Generative AI in the healthcare sector:
Virtual Clinical Trials: With the power of Generative AI, the possibility of conducting virtual clinical trials becomes more apparent. Simulating patient responses in a virtual environment could drastically reduce the time, costs, and ethical considerations associated with traditional clinical trials.
Hyper-personalized Medicine: By synthesizing and analyzing vast amounts of genetic and health data, Generative AI can pave the way for treatments tailored not just to a specific patient group, but to individual patients. This could result in interventions designed for a person’s unique genetic, environmental, and lifestyle context.
Advanced Prosthetics and Bioprinting: Generative AI can aid in designing more advanced prosthetics tailored to individual needs. Furthermore, in the realm of bioprinting, AI can help design and simulate the complex 3D structures of organs and tissues, potentially revolutionizing transplant medicine.
Enhanced Mental Health Support: Generative AI could be employed to create advanced therapeutic tools, such as chatbots capable of providing immediate, personalized mental health support, or simulating therapeutic scenarios for training and research.
Expanding Medical Knowledge: Generative models might be used to hypothesize the progression of unknown or less-understood diseases, potentially offering insights that can drive further research.
Improved Healthcare Logistics: Beyond direct patient care, Generative AI can be applied to optimize hospital operations, resource allocation, and patient flow, simulating various scenarios to determine the most efficient and effective strategies.
Real-time Disease Tracking and Prediction: Generative AI could be instrumental in predicting the spread of infectious diseases in real-time, simulating various intervention scenarios and helping authorities make informed decisions during outbreaks.
Ethical Medical Training: Training medical professionals often poses ethical dilemmas, especially when it comes to live practices. Generative AI can simulate real-life medical scenarios, allowing practitioners to gain experience without any risk to actual patients.
Revolutionizing Rehabilitation: Generative AI can aid in developing personalized rehabilitation protocols by simulating the body’s potential recovery trajectories, optimizing strategies for individual patients based on their specific needs and conditions.
Bridging Global Healthcare Gaps: Generative AI models, once trained, can be deployed anywhere, potentially offering high-level diagnostic and therapeutic suggestions in regions that lack expert healthcare professionals.
In essence, the prospects of Generative AI in healthcare are vast, promising a future where medical practices are more efficient, personalized, and data-driven. While challenges remain, especially in terms of ethics, data privacy, and technology integration, the potential benefits are substantial, heralding a new era in healthcare delivery and research.