The banking industry, long associated with tradition and conservatism, is undergoing a seismic shift in the age of digitization. One of the key drivers behind this transformation is Generative AI, a technology that has the potential to reshape the way banks operate, enhance customer experiences, and bolster security measures.
What does Generative AI Mean to Banking Industry?
Generative AI tools are reshaping the banking industry, with numerous fintech companies integrating advanced AI tools into their products and services, ushering in a wave of transformative innovations. These generative AI-powered solutions are streamlining processes, improving customer service, and enhancing fraud detection and risk management in the Banking sector. The utilization of cutting-edge technology is not only improving operational efficiency but also fostering a more seamless and secure Banking ecosystem for consumers and businesses alike. As this trend continues to gain momentum, we can anticipate even more remarkable advancements in the Banking technology landscape.
While we are in the early stages of the Generative Artificial Intelligence revolution, it is clear that various segments within the Banking industry are poised for significant transformation. Notably, retail banking and wealth management, SMB banking, commercial banking, and investment banking are among the verticals expected to undergo substantial changes. Incorporating Generative AI into the banking industry is anticipated to yield substantial benefits.
For example, this technology has the potential to deliver added value ranging from $200 billion to $340 billion annually if its diverse range of use cases were fully realized. Beyond the financial implications, the integration of Generative AI is poised to enhance operational efficiency, customer experiences, and security measures within the banking sector. As this technology continues to advance and find more applications, it is expected to play an increasingly pivotal role in reshaping the industry’s landscape and ensuring its competitiveness in the digital age.
Top 5 Use Cases of Generative AI in Banking
Generative AI has emerged as a transformative technology in the banking industry, revolutionizing various aspects of financial services. Here are the top seven use cases of Generative AI in banking.
Fraud Detection and Prevention:
Preventing fraud is a significant application of AI in the banking sector. To safeguard their operations, banks must prioritize data security.
Numerous banks operate extensive fraud prevention divisions, but these units can incur substantial operational and maintenance expenses, and, in certain instances, their efficacy may be limited.
Similar to how Generative AI is Employed in the Insurance Industry for fraud detection, banks can utilize it to monitor transactions based on criteria such as location, device, and operating system. This technology can then identify any deviations or unusual activities that do not align with established patterns. Subsequently, bank staff can assess the flagged suspicious behavior and determine whether it warrants additional scrutiny. This approach eliminates the need for manual transaction scrutiny, which is time-consuming and susceptible to human errors.
Furthermore, Generative Artificial Intelligence has the capacity to continuously extract synthetic data and enhance its detection algorithms, enabling it to stay abreast of the most recent fraud tactics. This proactive strategy empowers banks to foresee and preempt fraudulent activities before they occur.
Banks have the option to employ Generative AI to enhance security measures when users access their accounts. As an illustration, an AI-driven chatbot could prompt users to furnish supplementary verification, such as responding to a security question or undergoing a multifactor authentication (MFA) process.
The key takeaway is that banks have a multitude of avenues through which they can leverage Generative AI to enhance customer service, optimize operational efficiency, and fortify their defenses against fraudulent activities.
Credit Risk Assessment
Banks can harness Generative Artificial Intelligence for credit risk assessment, a critical element in averting financial crises and ensuring operational continuity. By analyzing historical data, Generative AI can identify and flag potential financial risks, offering early warning indicators that enable banks to proactively adapt and minimize potential losses.
Likewise, when it comes to credit scoring, banks engage in the task of assessing loan applicants. Rather than depending solely on conventional credit score components for gauging creditworthiness, banks can employ machine learning algorithms and AI to scrutinize extensive data from various origins, constructing a comprehensive financial profile of loan candidates.
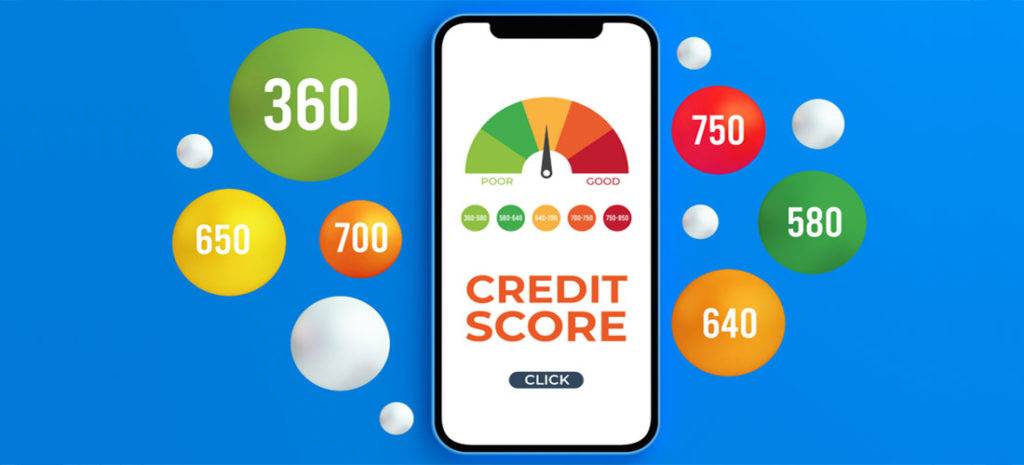
Generate Financial Predictions
Another advantage of AI training on past financial data is its capacity to assist banks in crafting financial projections and facilitating the creation of synthetic data.
Generative AI can discern patterns and connections within the data, and it can even conduct simulations grounded in hypothetical scenarios. Subsequently, this capability aids banks in assessing diverse potential results and devising corresponding plans.
In summary, Generative Artificial Intelligence leverages historical data to assist banks in making informed financial decisions for the future and generates synthetic data to conduct comprehensive risk exposure analyses.
Personalizing Marketing Strategies
Similar to all enterprises, banks must allocate resources to focused marketing efforts to distinguish themselves in a competitive landscape and attract new customers. However, achieving this goal is no simple task, as it demands extensive customer analysis and creative endeavors, entailing both significant costs and time investments.
Nonetheless, Artificial Intelligence can expedite your marketing endeavors by scrutinizing your customers’ preferences and online activities. Subsequently, it can categorize your leads into distinct segments, enabling the creation of diverse buyer personas. This approach empowers you to customize your marketing campaigns for various groups, aligning them with prevailing market conditions and trends.
Additionally, Generative AI can assist in the development of tailored marketing content and monitoring of conversion rates and customer satisfaction. This can be followed by the execution of A/B tests to assess the effectiveness of different approaches. Over time, these efforts will contribute to enhancing your marketing return on investment (ROI).
Enhance the customer experience and increase customer satisfaction:
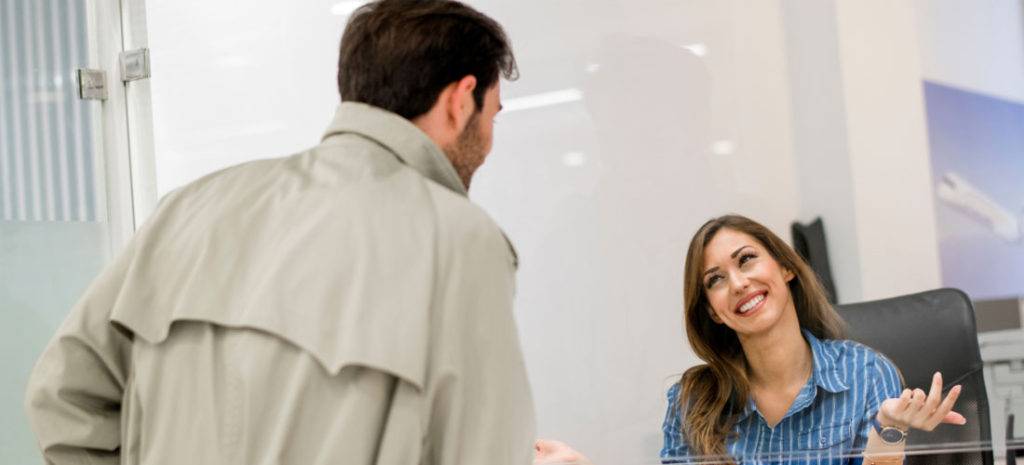
As a bank, your objective extends beyond acquiring new customers; it also involves retaining your existing clientele. Achieving this goal necessitates a continual focus on enhancing customer service and investing in the cultivation of a positive customer experience.
With the integration of natural language processing (NLP), Generative AI chatbots have the capability to offer round-the-clock, human-like customer support. They can address customer queries, furnish balance updates, facilitate transfers, and modify profile details. Moreover, they are proficient in handling more intricate inquiries as well.
Generative AI can simplify complex mathematical inquiries, such as determining a customer’s affordable mortgage amount, by swiftly producing a precise home buying budget when supplied with essential information like interest rate, down payment, and credit score. This enables it to assist customers in comprehending and discussing intricate financial calculations.
Additionally, Generative Artificial Intelligence has the capacity to provide education on various financial tasks and topics related to financial literacy, such as addressing inquiries about credit scores and loan practices, all while maintaining a natural and human-like conversational tone.
Furthermore, Generative AI can enhance the customer experience through personalization by leveraging customer data analysis to provide tailored product suggestions. For instance, it can propose a credit card that aligns with a customer’s spending patterns, financial objectives, and lifestyle, simplifying the selection process. This approach also enables Generative AI to support cross-selling and upselling efforts effectively.
Challenges and Limitations of Using Generative AI in Banking
Data Privacy and Security
One of the foremost concerns surrounding Generative AI in banking is data privacy and security. Banks deal with highly sensitive customer information, including personal and financial data. Any breach or misuse of this data can have severe consequences, including financial losses and damage to a bank’s reputation. Generative AI systems require access to large datasets for training, making it crucial to ensure robust security measures to protect customer information.
Regulatory Compliance
The banking sector is subject to strict regulations and compliance standards. Implementing Generative AI solutions must align with these regulations, which can vary significantly from one region to another. Ensuring that AI systems comply with laws like GDPR, CCPA, and financial industry-specific regulations is a complex task that requires careful consideration and constant monitoring.
Model Bias and Fairness
Generative AI models are trained on historical data, which may contain biases. These biases can be inadvertently perpetuated by the AI, leading to discriminatory outcomes in lending, customer service, or other banking processes. Banks must invest in ethical AI development practices to identify and mitigate bias, ensuring fairness and equal treatment for all customers.
Lack of Explainability
Generative AI models, especially deep learning models, are often regarded as “black boxes” because their decision-making processes are complex and not easily explainable. This lack of transparency can be a challenge in banking, where customers and regulatory bodies often demand explanations for decisions made by AI systems. Banks need to develop methods for making AI decisions more interpretable and justifiable.
Data Quality and Quantity
Generative AI models require vast amounts of high-quality data for training. In some cases, banks may struggle to obtain the necessary data due to limitations such as data silos, incomplete records, or data privacy concerns. This scarcity of data can hinder the effectiveness of AI models, making it essential to invest in data quality improvement efforts.
High Initial Costs
Implementing Generative AI solutions in banking requires a significant upfront investment in technology, talent, and infrastructure. Smaller banks or financial institutions with limited resources may find it challenging to compete with larger players in adopting these advanced AI technologies.
Technical Challenges
Generative AI models are computationally intensive and require robust hardware infrastructure for training and deployment. Banks need to invest in high-performance computing resources and skilled AI practitioners to manage and maintain these systems effectively.
Conclusion:
While Generative AI holds immense promise in transforming the banking industry by enhancing efficiency, improving customer experiences, and mitigating risks, it also comes with a set of challenges and limitations. Data privacy, regulatory compliance, model bias, explainability, data quality, high costs, and technical hurdles are among the key concerns that banks must address to successfully integrate Generative AI into their operations. Overcoming these challenges will require a concerted effort from the banking industry, regulators, and AI developers to ensure that the benefits of AI are realized while safeguarding the interests of customers and the integrity of the financial system.