Over the past few years, Generative AI has rapidly gained popularity in various industries. In Finance, it has been no different, as it has revolutionized the way businesses operate, and the financial industry is no exception.
Generative AI is ushering in profound shifts in the financial landscape, compelling the industry to rethink traditional operations and methodologies. This transformative technology is pushing the sector away from rigid deterministic models towards more adaptive, fluid systems that emphasize creativity and adaptive solutions.
As a result, professionals are being nudged towards interdisciplinary roles that combine financial acumen with AI understanding. Additionally, ethical and philosophical considerations are coming to the forefront, prompting debates about accountability, data integrity, and the very nature of decision-making in the age of machines.
The convergence of these factors has reshaped financial narratives, intertwining them with discussions on algorithms, data, and the broader implications of an AI integrated future.
An Overview of Generative AI in the Financial Services
The intersection of Generative AI and the financial services sector signifies a subtle yet profound evolution in the dynamics of an age-old industry. As the realm of finance gravitates towards modernization, the introduction of Generative AI subtly recalibrates the foundational ethos of financial practices. It challenges traditional deterministic notions, endorsing a system that’s more adaptable and fluid.
The emphasis shifts from structured predictability to a blend of creativity and innovation. Ethical deliberations find a more pronounced space as concerns around data integrity, accountability, and the philosophical implications of machine generated data come to the fore. Furthermore, professionals in financial services are witnessing a paradigm shift in their roles, necessitating a fusion of financial knowledge with a nuanced understanding of AI’s intricacies.
The discourse within financial circles is undergoing a metamorphosis, where conversations aren’t just about market trends but also the symbiotic relationship between algorithms and human decision-making. The engagement of Generative AI in this sector is not just a technological pivot but also a reflection of an evolving mindset and an industry in flux.
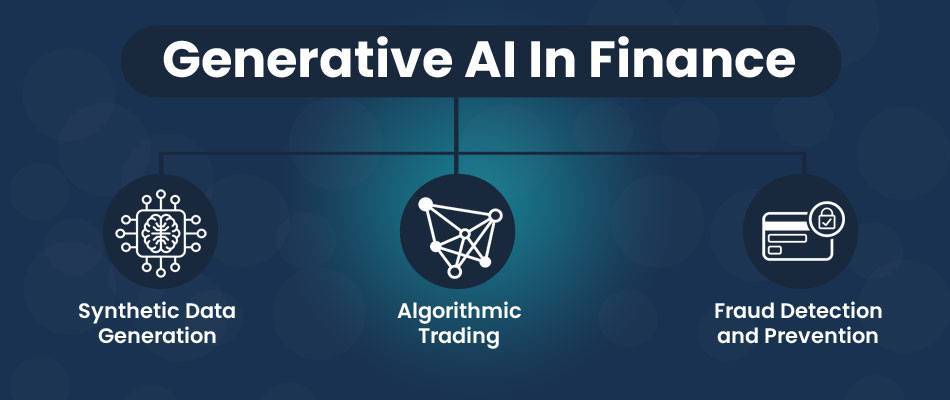
Prospects of Growth for Generative AI in the Insurance Industry
In today’s rapidly evolving technological landscape, the insurance industry finds itself at an exciting crossroads. Generative AI, a branch of artificial intelligence that specializes in creating new data from existing datasets, promises to usher in a new era of innovation and adaptability for insurers. The global market for Generative AI in financial services reflects this momentum. It is projected to grow from USD 847.2 Mn in 2022 to an astounding USD 9,475.2 Mn by 2032, marking a Compound Annual Growth Rate (CAGR) of 28.1% during the forecast period from 2023 to 2032.
Reimagining Claim Processing
Traditionally, claim verification and processing in the insurance sector have been prolonged endeavors. With Generative AI, there’s potential for a significant shift. By simulating diverse claims scenarios, insurers can hone their systems to fast-track and refine the claim validation process, translating to higher levels of customer satisfaction.
The Dawn of Personalized Policies
As the demand for personalized experiences continues to grow, the insurance industry can’t afford to lag. Generative AI offers the capability to analyze extensive data and tailor-make insurance policies to individual customer profiles, needs, and risk assessments. Such precision not only caters to customer preferences but allows insurers to maintain a well-balanced risk portfolio.
Elevating Fraud Detection Mechanisms
Fraud detection remains a pressing concern in the insurance world. Leveraging Generative AI, companies can simulate varied fraudulent activities. Such simulations empower systems to preemptively recognize and counteract suspicious actions, positioning insurers ahead in the battle against fraudulent claims.
Shifting Towards Dynamic Pricing
Economic fluctuations, environmental changes, and societal trends can all influence insurance pricing. Generative AI, with its ability to predict the ramifications of various scenarios on insurance needs, paves the way for dynamic pricing models. Such adaptability ensures both business sustainability for insurers and policy affordability for customers.
Redefining Risk Modeling
Generative AI holds the potential to bolster traditional risk assessment techniques. By generating comprehensive synthetic datasets, risk models can achieve heightened accuracy levels, leading to informed underwriting decisions.
Anticipating Challenges
While the prospects are bright, challenges are inevitable. Seamless integration of Generative AI into existing infrastructures, data privacy adherence, ethical considerations surrounding AI decision-making, and the need for workforce up skilling are potential obstacles that insurers need to navigate.
Various Generative AI Models Applied to the Financial Services
In the complex world of financial services, the application of advanced technologies has always played a pivotal role in driving innovation, efficiency, and customer experience. Among the most promising technologies of late is Generative AI, a subset of artificial intelligence that has been causing ripples across various sectors, including finance. This blog will delve into the various Generative AI models that have found applications within the financial realm.
1. Generative Adversarial Networks (GANs)
Perhaps the most renowned of all generative models, GANs operate through two neural networks- the generator and the discriminator – which work against each other. In financial services, GANs have found applications in:
Data Augmentation: They can generate synthetic financial datasets, which can be invaluable for training other machine learning models, especially when real data is scarce or sensitive.
Anomaly Detection: By training on normal transactional data, GANs can be used to detect anomalous transactions, which could be indicative of fraud.
2. Variational Autoencoders (VAEs)
VAEs are powerful tools in the world of generative modeling. Their structure allows them to compress data into a lower-dimensional space and then recreate it. Within the financial sector, VAEs are applied in:
Portfolio Optimization: By learning the underlying structure of asset returns, VAEs can aid in optimizing portfolio allocations.
Credit Risk Modelling: They can be used to learn the intricate patterns of credit default, helping banks predict and manage credit risk more efficiently.
3. Restricted Boltzmann Machines (RBMs)
Originally designed for binary classification tasks, RBMs have evolved as a prominent generative model. In finance, their applications include:
Feature Learning: RBMs can be used to identify and extract meaningful features from vast financial datasets, making them invaluable for tasks like stock market prediction or credit scoring.
Recommendation Systems: For financial institutions that offer a variety of products, RBMs can help in recommending the right product to a client based on their financial history and behavior.
4. Recursive Neural Networks (RvNNs)
RvNNs are adept at handling structured data, making them particularly useful in situations where relationships between data points are hierarchical or have a tree structure. Their main applications in finance are:
Financial Forecasting: They can process time-series data, like stock prices or foreign exchange rates, to predict future financial trends.
Document Analysis: RvNNs can analyze complex financial documents, contracts, or reports to extract essential information, aiding in tasks like compliance or risk assessment.
5. Transformer-based Generative Models
Models like OpenAI’s GPT (Generative Pre-trained Transformer) series, though primarily designed for natural language processing tasks, have potential financial applications:
Customer Service: They can generate human-like text, aiding in automated customer service or chatbots for financial advisories.
Financial Report Generation: These models can assist in auto-generating financial summaries, insights, or reports based on raw data.
Advantages of Generative AI in Financial Services
The incorporation of Generative AI into the financial services sector has brought about a wealth of advantages, including streamlining operations, enhancing predictive accuracy, and personalizing client interactions. Here are some of the prominent benefits:
1. Enhanced Data Augmentation: Generative AI can produce synthetic data resembling real financial datasets. This capability is invaluable, especially when genuine data is scarce, sensitive, or expensive to obtain. By augmenting real data with synthetic data, financial models can be trained more robustly, ensuring they generalize well in real-world scenarios.
2. Improved Risk Management: Generative models can simulate various economic conditions, market shifts, and customer behaviors. By understanding these scenarios, financial institutions can better forecast potential risks and create strategies to mitigate them, thus fortifying their risk management systems.
3. Advanced Fraud Detection: Generative AI, when trained on normal transactional data, can highlight anomalies or unusual patterns. These irregularities, once detected, can be flagged for further investigation, enhancing fraud detection systems and safeguarding both institutions and their clients.
4. Tailored Financial Products: With the power to analyze vast amounts of data and predict future trends, Generative AI can assist financial institutions in designing customized financial products or investment portfolios. These tailor-made solutions cater to individual client needs, preferences, and risk appetites, leading to improved customer satisfaction.
5. Operational Efficiency: Automated and more accurate decision-making processes facilitated by Generative AI can streamline various operations, from loan approvals to asset management. This automation not only speeds up processes but also reduces the margin of error.
6. Dynamic Pricing Models: By simulating various market conditions, Generative AI helps institutions develop pricing models for their products that can adapt to changing market dynamics, ensuring that their offerings remain competitive and relevant.
7. Personalized Customer Interactions: Generative models, especially those adept at natural language generation, can power chatbots and virtual financial assistants. These AI-driven platforms can engage customers, address queries, and provide advice, offering a personalized touch without human intervention.
8. Innovative Investment Strategies: By analyzing market trends, global events, and historical data, Generative AI can assist in devising novel investment strategies, helping investors find opportunities they might have otherwise overlooked.
9. Reduced Reliance on Historical Data: While historical data remains vital, Generative AI models can simulate potential future scenarios, making it possible for financial institutions to make decisions that are not solely anchored to past events.
10. Regulatory Compliance: By analyzing complex regulatory documents and changes, Generative AI can ensure that financial institutions remain compliant, reducing the risk of legal complications or penalties.
Applications of Generative AI in the Financial Services
The evolving landscape of financial services is marked by a consistent push towards digitization, precision, and customer-centricity. Among the technologies paving the way for this transformation, Generative AI stands out prominently. This form of artificial intelligence is making waves by generating new data patterns and predicting outcomes based on existing datasets. Here’s a closer look at how Generative AI is being applied in the realm of financial services.
1. Data Synthesis
One of the primary challenges in the financial sector is obtaining ample quality data for various analyses, especially when dealing with constraints related to privacy or regulation. Generative AI offers a solution by creating synthetic datasets that closely resemble real-world financial data. This not only aids in model training but also ensures there’s no breach of data confidentiality.
2. Fraud Detection
The capability of Generative AI to simulate diverse transactional patterns positions it as a potent tool in the fight against financial fraud. By training these AI models on legitimate transaction data, they can be fine-tuned to detect anomalies, thereby strengthening fraud detection mechanisms.
3. Portfolio Optimization
Investment strategies are increasingly reliant on data-driven insights. Generative AI, with its ability to simulate a myriad of market scenarios, serves as a valuable asset in crafting optimal investment portfolios. By understanding the performance of different asset combinations under various conditions, it offers insights that can be pivotal for wealth managers.
4. Financial Forecasting
Predicting the financial future, be it stock price movements or interest rate changes, has always been a mix of art and science. Generative AI adds more weight to the scientific side, processing vast amounts of historical data and producing forecasts that help in more informed decisionmaking.
5. Personalized Financial Products
The demand for tailored financial solutions is on the rise. Generative AI can analyze individual behaviors, needs, and preferences to aid in the design of bespoke financial products. Such a tailored approach ensures that clients receive services uniquely suited to their requirements.
6. Credit Risk Assessment
Generative AI’s prowess in simulating financial behaviors provides a robust platform for predicting credit risks. By analyzing patterns and generating potential financial scenarios, it assists institutions in making informed lending decisions, ensuring a balanced risk-reward equation.
7. Regulatory Compliance Simulations
Adhering to evolving regulations is a challenge for any financial institution. Generative AI can create various compliance scenarios, offering foresights into potential regulatory challenges. This preemptive approach ensures firms are always a step ahead in compliance matters.
8. Real-time Market Simulations
Understanding market reactions in real-time can be invaluable, especially for traders and institutional investors. Generative AI’s ability to instantly simulate market responses to various events, from corporate announcements to macroeconomic changes, offers actionable insights to
market participants.
Generative AI in Financial Services: Real-World Examples
The financial services industry, known for its intricate data structures and dynamic market scenarios, is constantly on the lookout for innovative solutions. Among the myriad of technologies available, Generative AI stands out, offering transformative potential. Let’s explore some real-world instances where financial institutions have tapped into the power of Generative AI to redefine their operations and customer engagement.
1. JPMorgan’s LOXM Execution Algorithm
One of the world’s leading financial services firms, JPMorgan, incorporated Generative AI into their LOXM execution algorithm. The system uses historical data to generate new trading strategies. By simulating thousands of trading scenarios, LOXM helps traders optimize their order execution, ensuring that trades are placed efficiently while minimizing market impact.
2. Numerai’s Crowdsourced Hedge Fund Model
Numerai, a hedge fund structured by data scientists worldwide, uses generative models to transform real-world stock market data into abstract numerical data, which is then shared with a network of anonymous data scientists. They build predictive models and share them back with Numerai. The firm then aggregates these models into a meta-model to make its market bets. It’s a unique approach that combines the power of crowdsourcing with Generative AI.
3. American Express’ Fraud Prevention
To protect its vast network of cardholders, American Express uses Generative AI to simulate fraudulent transaction patterns. By understanding the tactics and techniques of fraudsters, they’ve managed to enhance their detection algorithms, reducing false positives and catching fraudulent activity with increased precision.
4. Morgan Stanley’s Next Best Action System
Morgan Stanley, another global financial juggernaut, leverages Generative AI in its “Next Best Action” system. The AI-driven tool analyzes vast quantities of data to generate investment suggestions and potential strategies for financial advisors. By assimilating information on market conditions, client portfolios, and investment trends, the system aids advisors in making more informed, timely decisions.
5. Two Sigma’s Investment Models
Two Sigma, a hedge fund known for its tech-savvy approach, integrates Generative AI to construct sophisticated investment models. By simulating countless market scenarios based on historical and real-time data, they’re able to glean insights into potential market movements and adjust their strategies accordingly.
Conclusion:
Generative AI is changing the landscape of finance by automating several tasks, reducing costs, improving efficiency, and providing better investment advice. With the help of Generative AI, companies can make better investment decisions, manage risk better, and provide personalized investment advice to customers.
As Generative AI continues to evolve, it will likely become even more prevalent in the financial industry, making it easier for companies to operate and providing customers with better investment advice.